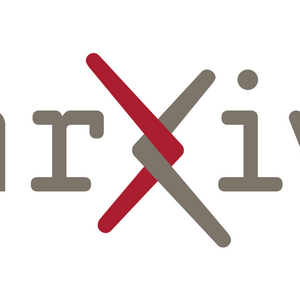
Exploring the Limits of Transfer Learning with a Unified Text-to-Text Transformer
- Paper
- Aug 28, 2020
- #MachineLearning #Naturallanguageprocessing
Transfer learning, where a model is first pre-trained on a data-rich task before being fine-tuned on a downstream task, has emerged as a powerful technique in natural language proce...
Show More